Meshing Data Science With Advanced Manufacturing
With Industry 4.0, it is becoming more evident that any advancements in the physical realm are becoming ever more dependent on the digital world, authors say.
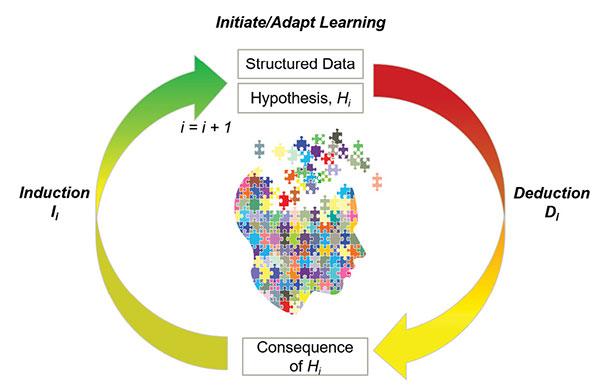
The Iterative Learning Process. Image courtesy of Matthew Sloane, Ph.D., and Frank Gagliardi, Ph.D.
Latest News
March 15, 2021
By Matthew Sloane, Ph.D., and Frank Gagliardi, Ph.D.
Advanced manufacturing and its associated technologies, such as the Industrial Internet of Things (IIoT) that encompasses hardware and software, are revolutionizing the manufacturing processes aimed at optimizing product production.
Within this realm, we find representational digitization as the prime mover, and data the fuel for its models, code and automata. As we enter this realm, which some call Industry 4.0, it is overly evident that any advancements in the physical realm are becoming ever more dependent on the digital world.
Additive manufacturing (AM), a subtechnology of the advanced manufacturing realm, exemplifies this dependency. It is inherently dependent on digitization for the modeling and construction of products. Recognizably, it is the vast amount of data produced throughout the build process and during post-build testing that calls for the integration of data science techniques, which hold the keys to efficiently unlock AM’s power. The proper and rapid analysis of data leads to greater returns on investment and provide a means to incorporate the mass commercialization of additive technology in traditionally regulated industries.
An AM strategy rooted in extracting information from rigorous data analysis and applying it as knowledge enables design and forms the foundation for robust qualification and certification processes. Such well-structured knowledge is an essential component for producing higher quality-lower cost products and services.
A Methodical Approach to Data Analysis
The cautionary portent here is that engineers and managers alike may be tempted to apply “advanced analytics” immediately in an unstructured effort to learn quickly about new, data-rich manufacturing processes.
From a sound business perspective, this is ill-advised. It is incumbent that these engineers, managers and leaders develop and follow a structured, methodical approach to data analysis and analytics. This approach must encompass structures and tools that will lead to better decision-making by transforming specific data to actionable knowledge.
Minimally, a four-step process should include: identify the specific problem and requisite data needed to solve it; apply an iterative learning process to gain an understanding of the situation; model the phenomena in consideration; and use gained knowledge to guide their courses of action.
Manufacturing-centric teams must prepare a strategy early on for identifying, preparing and incorporating pertinent data into their AM projects. Too often, teams ignore the importance of a well-planned strategy and seek answers solely from haphazardly conceived data. Prior to starting a project, teams must identify the specific problem they seek to solve, act in a hypothesis-driven manner and drive intensely at collecting and structuring the data necessary to prove or disprove their hypotheses.
Team engineers, data scientists and managers that prioritize this deliberate strategic approach to data creation and data wrangling will reap the benefits of their efforts. It is important that the stakeholders understand that the type and quality of AM data must be transformative, and not merely based on abundance.
With properly structured data in hand, the teams can seek solutions by applying a hypothesis-driven iterative learning process. The iterative (and adaptive) learning process (Fig. 1) initiates with use of thorough descriptive statistics and visual representations.
The data representations set the basis for analysis with inferential statistics and cycles of deduction and induction leading to final hypotheses regarding the manufacturing processes. These hypothesis types will prove to be of paramount importance and foundational for the teams as AM continues to mature and gain a foothold in the advanced manufacturing industry.
Robust hypotheses allow team members to move to transform what was once seemingly disparate data to a whole representation of the AM process. This is essential because the AM process is inherently complex and data-rich. As the team moves to the modeling phase, their continuous improvement goal should be an effort to optimize the model and represent the process in the simplest, most accurate way.
For example, the model may include an array of independent variables in multiple linear regression analyses to account for factors that influence the dependent variable (or outcome of interest).
At some point, the team will certainly understand the trade-off between complexity and cost if they employ additional independent variables that fail to enhance the model significantly. However, complete teams comprised of engineers, data scientists and managers who grasp this concept and holistically model the process with a data and physics mindset will run truly successful AM projects.
A deliberate and structured approach to incorporating data science with AM will yield models that set a realistic expectation for future manufacturing process behavior. Equipped with this knowledge, engineering and manufacturing teams will be able to design and deliver better products faster. With this proposed discipline and rigor in evaluating data, AM technologies may realize their true transformative power.
Matthew Sloane, Ph.D., and Frank Gagliardi, Ph.D., are the principal investigators for the Advanced Manufacturing Cell of the Quality Engineering and System Assurance Directorate at the U.S. Army Combat Capabilities Development Command - Armaments Center.
Subscribe to our FREE magazine,
FREE email newsletters or both!Latest News
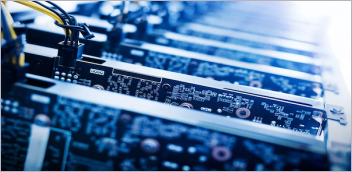
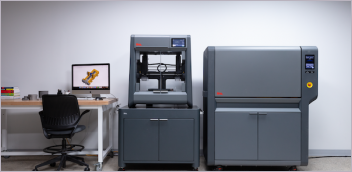
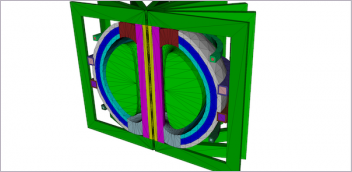
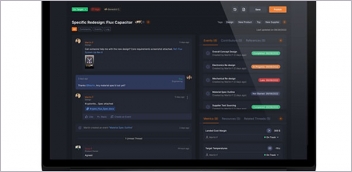