
Movements captured in Xsens suits can be imported into Siemens Tecnomatix Jack and Jill software. Image courtesy of Siemens PLM Software.
Latest News
June 1, 2015

With characteristic English wit, Samuel Taylor Coleridge quipped in a verse that, as a general rule, he would concede, “every poet is a fool … but every fool is not a poet.” In light of the current rush to embrace the Internet of Things (IoT), both for its popularity and its legitimate appeal, it’s worth making a similar distinction. Every IoT device is connected, but every connected device is not IoT-enabled.
Connecting a vehicle or a thermostat to the Internet doesn’t take a lot of effort (or imagination, for that matter). IoT demands something more. An IoT product is greater than the sum of its parts. Streaming weather and traffic data from the cloud to the dashboard doesn’t make the vehicle “smart.” A thermostat that uploads hourly temperature readings to the cloud isn’t adding any value. But if the vehicle can detect potential traffic jams and suggest alternate routes, or if the thermostat can turn itself down when the occupants are absent, you might say the product has been infused with IoT magic.
Strictly speaking, that magic is no sorcery. It’s programming logic, pattern recognition and machine learning, aided by sensors and electronics. The ghost in the machine is not in the geometry of the product, the circuits or the lines of code. It’s in the intelligent manner in which all these components work in synchronicity to produce the illusion of autonomy.
The emerging IoT technologies featured here suggest the emphasis in product development is shifting from geometry to data acquisition and analysis, and from geometry-based simulation to sensor-driven simulation.
Your Factory is Watching and Listening to You
Adrian Jennings cares about what seems like a ridiculously small amount of time: six seconds. “In a high-volume manufacturing facility, you only get about 60 seconds to 90 seconds to work on a vehicle that comes into your station. So if you have to take six seconds—roughly 10% of the allotted time—to scan the car each time one comes up for work, it adds up,” says Jennings, vice president of Manufacturing Industry Strategy at Ubisense.
The point of scanning the product is to identify the vehicle, so you can be sure you’re performing the right operation on it. So Jennings and his colleagues at Ubisense developed a technology that could automatically identify the car, reclaiming those precious six seconds.
“Think of it as indoor GPS,” Jennings says. “You have active RFID (radio frequency identification) tags that are roughly the size of a deck of cards installed on your assets and tools, and sensors installed along the assembly line. The tags are always pinging, and the factory is listening.”
With this setup, the factory knows, for example, that car 12 has entered workstation 100, and that tool x is present in the same workstation. This location intelligence ensures that you’re using the right tool on the right asset, applying the correct torque to perform the designated task. Conversely, if you pick up the wrong tool, it would refuse to complete the operation because the factory knows the tool is inappropriate for the job at hand.
“This eliminates the need to physically fasten tools to a specific workstation with cords. Your tools can be cordless,” says Jennings. Up to this point, the technology works to increase efficiency and error-proofing the assembly line, but Jennings has bigger visions. “One of the machine-learning opportunities is in accessing line performance and crisis prediction,” he says.
There are telltale signs of impending troubles, detectable in the patterns of delay in workstations. “Assembly lines are like traffic flows. As soon as someone slams on a brake, everyone else behind slams on it a little harder,” says Jennings. “So if someone is starting the process a few seconds behind every time, it could be a sign the line is heading for a crisis. That’s something an AI-equipped factory can recognize on its own.”
Your Virtual Workers Reporting for Work
Ubisense’s Jennings points out that, contrary to what you might have heard, the automotive assembly process is highly manual, driven by humans rather than robots. That’s why digital human models like Siemens PLM Software’s Jack and Jill, part of the company’s Tecnomatix process simulation software, could offer new opportunities.
“Every now and then you see one person who is just way better than everybody else in the assembly line,” says Jennings. “Is there something he or she is doing differently from the rest? If you can capture his or her movements, there’s a chance it can be replicated.”
Replicating real human motions with digital models has been perfected by animators and game developers. The use of motion-capture suits propels the data-acquisition process to new heights. Xsens, a Netherlands-headquartered 3D motion-tracking technology developer, is hoping to improve the process with its gyroscopic suits. Xsens is a partner of Siemens PLM Software. The two work to make it possible to bring movements captured by actors in Xsens MVN BIOMECH suits to Siemens’ Jack and Jill.

“The suit from Xsens is a good example of sensor fusion. It involves gyroscopes, accelerometers, and magnetometers. You can put on that suit and start walking around,” says Ulrich Raschke, director of Tecnomatix Human Simulation products, Siemens PLM Software. “It’s a self-contained tracking system. With motion capture using markers and cameras, you’d have to worry about camera occlusion (the camera’s inability to see the subject with markers). With this suit, you don’t have to worry about that.”
Typical use of Xsens technology may involve, for example, having an actor perform or mimic a particular assembly operation or enter and exit a mockup vehicle. Ergonomists could analyze the movement captured in Siemens’ software to understand the stress and strain involved. If needed, the assembly process or the vehicle’s doors could be redesigned to allow a much more natural movement.
Ergonomic analysis has emerged as an important part of manufacturing process simulation. “When you’re designing something new, you don’t know how people will approach it. When planning for wire-harnessing for a plane, you may assume people will hold the wire and use a kneeling posture to do it. But then when you see the subject performing the task, you might realize people have a tendency to do it in differently. The data from the suit can be streamed directly to the Jack software in real time. So while the actor is acting out a task to see if he or she can reach for certain things, the software can calculate if, over a period of time, this action exposes the human employee to injury risks,” says Raschke.
The latest enhancement in Ubisense technology adds head tracking, which offers the chance to derive new insights from captured movement. “If somebody is wearing a head-mounted display that includes tracking, we now know where they are, what machine they are standing in front of and what information might be useful to present,” says Raschke.

Your Virtual Sensors Ready to Go
Other executives are trying to bring together elements that previously existed in separate spheres. “Imagine a CAD model with sensors in it,” says Brian Thompson, senior vice president for PTC Creo.
A CAD model made of pixels and polygons cannot accommodate real sensors, but Thompson and his colleagues have worked out a way to stream real-time data coming from ThingWorx, PTC’s IoT application development platform, to a CAD model. By connecting the field data from the sensors to ThingWorx, Thompson and his team accomplish what they set out to do.
“As you start collecting data on a product and learn about it, your PTC Creo model of the product could become the digital twin of the physical prototype,” says Thompson. “We think of that as a 3D digital twin [of the physical unit] equipped with virtual sensors.”
In an augmented-reality display, the CAD model, the real-time video feed of the product in action (say, a bike in motion with a rider), and the raw data from the experiment (such as speed, forces and pressure) could be consolidated. It may also be possible to incorporate real-time stress analysis results into the same environment, taking the concept of simulation to new heights. “The ability to view the digital twin in conjunction with raw data from a real-time event lets you see instantly whether you’ve overdesigned or under-designed something,” says Thompson.
Thompson and his team have also implemented certain software-driven analysis and alerts. For example, the software can recognize from a real-time data feed that a bike’s front and rear wheels are spinning at different rates—a potential risk factor. “It’s not just about reporting data,” he says. “It’s meant to give you additional insights.”
Thompson and his colleagues said: “We’re committed to make this connection [between ThingWorx and PTC Creo] work. How would this manifest in our product? How will our customers implement it or deploy it? That’s up for discussion.”
The Internet of ‘Think’
Machine learning or artificial intelligence (AI) refers to devices that can not only sense and react but “think” and “learn.” Through programmed logic, they can act based on what they perceive. Over time, their ability to act without user intervention grows. Zach Supalla, founder and CEO of Spark, has some insights into where the thinking and learning happen.
“You have sensors that are usually pretty dumb. You also have actuators that are also dumb. Neither of them are usually doing the thinking. Something else provides the logic. Often you don’t want that to be in the device, because it’s complex, changes all the time and requires lots of resources. So it makes more sense for that to be on the server,” Supalla says. In other words: the cloud.
Take, for instance, a farm equipped with weather sensors and sprinklers. The sensors detect conditions, but do not react; the sprinklers can react to command, but do not think. The two enter the realm of IoT when something else provides the logic to activate the sprinklers if the ground is dry and no rain is expected.
“Machine learning happens when you turn that logic into an abstraction, remove it from the hardware, and make it grow in complexity over time,” says Supalla. An IoT device like the Nest learning thermostat, he pointed out, would do the learning in the cloud, not on the device itself.
Spark offers IoT development kits—the Spark Core (Wi-Fi), the Photon (Wi-Fi), and the Electron (2G/3G cellular)—for prototyping connected devices. It also offers private cloud solutions, a secure space for your devices to think, as it were.
Since the real-time and historical data collected from sensors serves as the source for the device’s thinking and learning, some product designers may have to tackle the Big Data headache. “If you’re storing temperatures, the two-digit integer doesn’t require a lot of room,” Supalla explains. “But if you collect temperature for every second, even though the second-to-second temperature variation is minimal, you could unnecessarily create a Big Data problem. Maybe you just need to know temperatures for every five minutes to make effective decisions.” The smart approach, he says, is to identify and collect only meaningful data.
For CAD users who focus on the geometry of the products, Supalla says it is critical to think of making room for sensors and actuators in the products, along with the means to power them. Perhaps less obvious, they may need to consider whether the device’s wireless transmission and reception might be hampered by nearby objects. That requires not only thinking about the geometry and shape of the product, but also a good understanding of the field conditions for deployment.
On the Eve of an Evolution
Siemens’ Raschke thinks the manufacturing industry is on the cusp of something big. All the technology pieces are in place, awaiting a major transformation. “We already hold much of the information about the machineries in the plant, about the movement of the people, and so on. We’re on the doorstep of connecting those data and wearable devices,” he says.
Spark’s Supalla cautions against a simplistic interpretation of IoT. “AI doesn’t necessary need automated robots. Think of Uber, for example. The user transmits their locations and requests cars [from an app on a mobile device]. They don’t have to call up a taxi service to order a car. Some part of the system is automated, but that small part makes a huge difference,” he says.
The momentum for the IoT and the zeal to deploy it may lead some manufacturers to tunr to autonomous or semiautonomous robots. Such adaptation will surely add speed and efficiency to the operation, but doesn’t exploit the full potential of the IoT. Perhaps the inherent paradox in the term “Internet of Things” is that it requires looking at products not as things—but as pieces that produce an overall experience.
More Info
Subscribe to our FREE magazine,
FREE email newsletters or both!Latest News
About the Author

Kenneth Wong is Digital Engineering’s resident blogger and senior editor. Email him at [email protected] or share your thoughts on this article at digitaleng.news/facebook.
Follow DE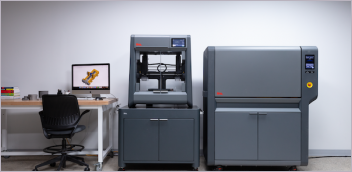
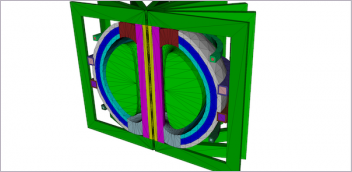
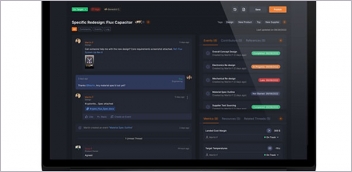
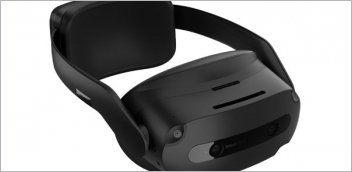