Should Engineers Embrace Data Science?
Increased adoption of machine learning and AI in design and simulation will require a higher level of understanding by engineers.
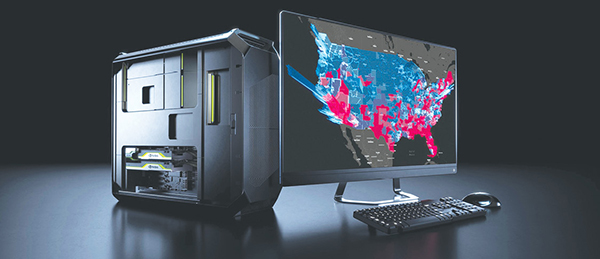
The NVIDIA Data Science Workstation specification is primarily used by vendors to create desktop units, but the hardware and software are also available for mobile workstations. Image courtesy of NVIDIA.
Latest News
March 9, 2020
Demand for data scientists is expanding as more companies embrace artificial intelligence (AI) and machine learning in their design processes. This shift has meant that engineers need a greater grasp of data science concepts in order to effectively integrate these technologies into the products they design, as well as their own processes. Engineers can benefit from training in this specialty to better take advantage of the technology.
“Hiring deep learning engineers can be a challenge, as the field has only recently become so big,” says Leslie Nooteboom, CDO and co-founder of Humanising Autonomy. “The level of expertise required depends on the application. If the engineer will have to develop new model architectures, it will require several years of experience. If the engineer will need to retrain existing models with new data, less seniority is required. On the design engineer side, an understanding of the deep learning development process and its limitations is the least they should be capable of.”
While data scientists bring a wealth of expertise to an organization that is integrating AI into its operations, these scientists lack the domain expertise needed to understand which data is most valuable and where to best apply data analytics to solve a given problem. Systems and software engineers must work closely with them to ensure the success of these projects. To do so, however, they need a better understanding of data science and how it can improve their designs.
In addition, deep learning and AI can enable more efficient integration of computationally expensive component models into system simulation models by replacing these component models with high-fidelity response surface models (RSMs) or functional mock-up units (FMUs). Other potential applications include the analysis of complex computational fluid dynamics (CFD) images to locate specific features such as turbulence, or the evaluation of a high number of different designs for feasibility.
In many organizations, however, the data science and engineering teams are heavily siloed. This can make it difficult to address some of the requirements in building a data-driven product. While data scientists can build the proper machine learning models, they not have a full view of the elements needed to build the model—what raw data will be required, what problems need solved, and how the model fits within the desired user experience.
Predictive Maintenance: Marrying Data Science and Engineering
One of the areas that has shown early promise when it comes to marrying engineering and data science is in the realm of predictive maintenance. Equipment downtime is an increasingly costly problem, so designers and manufacturers are looking for ways to better anticipate product failures and address them in advance.
AI and machine learning are excellent tools for these applications. By analyzing existing failure data, and incorporate data from large numbers of failure simulations, predictive maintenance tools can be developed that can issue alerts when conditions indicate an imminent failure. As more data is collected, these tools can become even more accurate and reliable.
At MathWorks, the company is building tools that can help both data scientists and engineers utilize artificial intelligence to create predictive maintenance applications, says Philipp Wallner, industry manager at MathWorks.
While some companies are hiring data scientists to do this work, others are attempting to train engineers on these same tools. In some organizations, the engineers and data scientists have to work more closely together to ensure success in these development projects.
“What we’ve seen as a successful approach is the engineers doing the pre-work like getting the data into MATLAB, and then running different machine learning algorithms over that data and checking plausibility,” Wallner says. “This is where the domain experts are better suited, because of their background.”
MathWorks offers training for engineers to help them better understand the data science capabilities in the tools. “We have customers that haven’t hired any data scientists, and are still successful with predictive maintenance applications,” Wallner says. “However, the most successful are the companies in which the engineers and data scientists are working together.”
“There are a lot of different ways to implement AI techniques, but most of them are targeted at data scientists—people who have a good or very deep mathematical or statistics background,” Wallner continues. “What we see in our industry, is that it is not only important that data scientists can work with the tool they use to implement AI applications, but it is also important that domain experts or engineers (who have a good understanding of the equipment itself) are able to work with those tools. That’s why we are trying to create an environment that helps engineers as well as data scientists to do AI.”
Engineers also need access to the compute power required for these analytics tasks. NVIDIA announced its NVIDIA-Powered Data Science Workstation specification last year. “Data science is one of the fastest growing fields of computer science and impacts every industry,” said NVIDIA founder and CEO Jensen Huang when the specification was announced. “Enterprises are eager to unlock the value of their business data using machine learning and are hiring—at an unprecedented rate—data scientists who require powerful workstations architected specifically for their needs.”
To learn more about deep learning and data science in the engineering sphere, visit NVIDIA’s GTC 2020 conference (now an online event).
More Dell Coverage
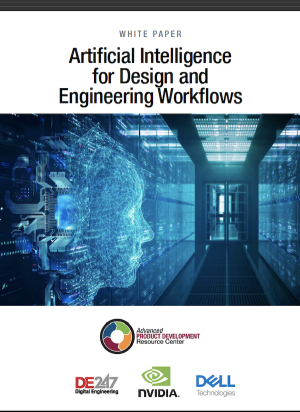
More MathWorks Coverage
More NVIDIA Coverage
Subscribe to our FREE magazine,
FREE email newsletters or both!Latest News
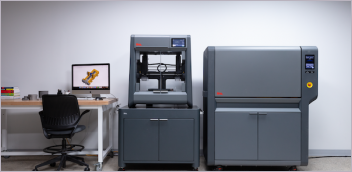
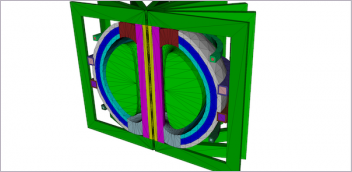
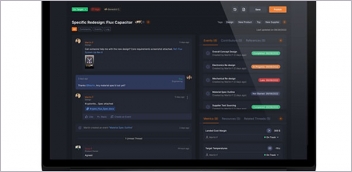
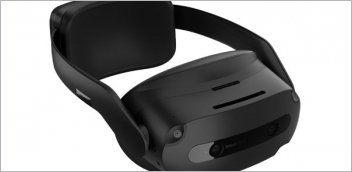