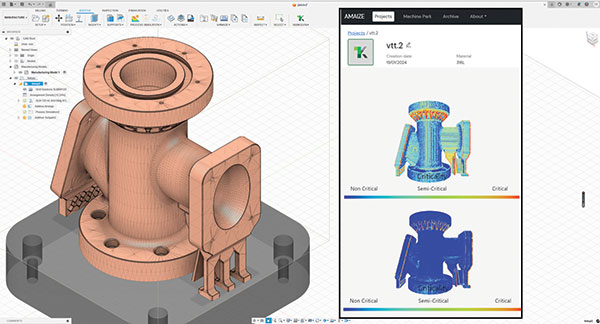
1000 Kelvin’s AMAIZE is now integrated in Autodesk Fusion, enabling engineers to analyze and optimize their AM designs seamlessly. Image courtesy of Autodesk and 1000 Kelvin.
Latest News
April 5, 2024
Additive manufacturing (AM) emerged as a rapid prototyping technology in the late 1980s. Over the last four decades, hardware and software makers have accumulated a good understanding of what prints well, and what doesn’t, allowing them to integrate the knowledge into their print preparation software to nudge users away from designs that are destined to fail.
The next step in this evolution is the incorporation of AI (artificial intelligence). Some leading design for AM (DfAM) software makers are already hedging in this direction. In this article, we speak to them to understand how the move will change AM applications.
Let the AI Sort it Out
Materialise provides AM services and software solutions focused on industrial and healthcare applications. For Bart Van der Schueren, chief technology officer of Materialise, the dental field is an area where he can clearly see the benefits of AM.
“You have bridges, partial bridges and crowns. With AI and machine learning, it’s now possible to recognize these objects from their geometry,” he says.
This artificial intelligence (AI)-based shape recognition has an impact in automating various aspects of print preparation, he points out. “Each type of topology has a specific orientation that’s best for a machine, has a specific support structure it needs, and requires specific print parameters,” he says.
With AI-based object recognition, “the operator can simply drag and drop these topologies onto the platform and let the AI classify them. Similarly, in [computed tomography (CT)] scans, AI can recognize the type of bones based on the scan data,” he explains.
Monitoring Defects
Another area where computer vision adds value is in the print process itself. AI programs can perceive, detect and record much more than the human eye. “In heavily regulated industries like aerospace, the cost of printing is only about 30% to 50% of the production cost. The rest goes to quality control.
“AI can already monitor and identify components that are not good enough, those that have internal defects that are not visible to the naked eye. That saves a lot of quality control efforts during the post-processing of the components,” he explains.
Today, Van der Schueren doesn’t think AI programs are mature enough to reliably predict print failure before the printing process, but it’s only a matter of time.
“We can start using the information about defects gathered during the printing process to understand the root causes, then combine anomaly detection with simulation technology. This will help to see correlations between simulation results and defects,” he says.
He believes more calibration is needed to fine-tune the existing AM simulation models so they can accurately predict where and how print failure might occur. A hurdle that stands in the way of better AM simulation models, Van der Schueren noted, is the lack of data.
“For machine learning to work well, we need to have lots of data. But what we often see is that users don’t want to share their data because they consider it their own proprietary information,” he notes.
Failure Mechanism
Simulation software maker Ansys’ portfolio includes the Ansys Additive Suite, specifically tailored to AM projects.
“The extremely high-fidelity metal Laser Powder Bed Fusion (LPBF) Thermal Strain simulation within the Additive Suite capability in Ansys Mechanical can simulate based on every individual scan vector in a build,” says Christopher Robinson, senior product manager at Ansys.
Simulation is the key to understanding the thermochemical activities involved in 3D printing, and also to understanding the failure mechanisms.
“Failure is a word with a broad definition. Many parts are built to completion, but they still fail to meet performance, surface or geometric tolerances,” Robinson explains.
He notes that many mechanisms can lead to failure, such as recoater interference, part or support cracking, excessive deformation, thermal overheating, demarcations or shrink lines, misunderstood grain structures, porosity, surface roughness, and others. There are various types of simulation solvers that can be used to solve such challenges, ranging from structural, thermal, fluid and discrete element solvers to inference-driven AI models based on simulation or empirical data, according to Robinson.
A Way to Train Your Own AM AI
There are general rules applicable to all AM geometry. For example, thin walls will likely collapse during printing and certain overhanging features will need support structures during print. Even without AI, many print-preparation tools can recognize these problems from the CAD model and recommend fixes. But the success or failure of a job depends on something else besides geometry: the print hardware.
Because each printer has its own unique print procedures, from thermal management to powder deposit, it affects the printed object differently. A design that prints successfully in one machine might fail in another. A design that distorts very little in one machine might warp beyond the usable point in another. Therefore, print failure prediction based on hardware would require additional AI training.
To solve the dilemma, “It’s important that, as a company, we don’t just give pretrained models to our customers, but also give them a way to easily train the models further with their own data,” Van der Schueren suggests.
Robinson points out, “Even within the same process, if you switch materials or machine vendors, or vary processing parameters, or have different airflow within the machine, or different energy source profiles, then it is possible to run into situations where buildability is not consistent.”
With the launch of SimAI early this year, Ansys is hoping to serve the same need.
“AI integrated with simulation can help to make ultra-high-fidelity simulations available to users that will take the next step beyond generic predictions. For example, you have the option of simulating based off an LPBF build file, where you can really start to understand machine-specific details,” says Robinson.
Pretrained to Predict
Omar Fergani, CEO of 1000 Kelvin, has a good understanding of failure mechanisms. He was an AM application engineer in his early career. He did his Ph.D. on residual stress at The Georgia Institute of Technology because he was, as he put it, “fascinated with solving this problem.”
1000 Kelvin describes its offering as a “one-stop shop AI software to predict near real-time print issues, perform corrections, and generate industrial-grade, ready-to-use print files specific to your machine.”
Fergani explains, “Instead of giving a finite element analysis (FEA) software product to an engineer to solve their AM problems, we decided to take the FEA solvers, train machine learning systems, make them extremely powerful in terms of speed, effectiveness, optimization and then package them as a product for the engineers.”
Due to the time it takes to faithfully simulate AM events using traditional finite element analysis, the use of machine learning models is inevitable, Fergani points out. “If you want to simulate part-level deformations, it only takes a few hours. But if you want to simulate it at the melt pool level, typically it takes two hours to compute 2 millimeters of toolpath, and printing a part usually requires thousands of kilometers of toolpath …,” he calculates.
Some laser- and toolpath-related changes that occur in the print process are simply too complicated to simulate, in his view.
“But deep learning or AI models can actually make predictions of these things,” he says. “Every time we do a prediction for thermal optimization, it’s machine-specific and material-specific. It’s not an issue for us because we are computationally efficient. It takes just a few minutes to do this analysis.”
Once this step is possible, the next step becomes much simpler. “Now, you can ask our software to give you a new print strategy or print recipe to avoid the failures,” he says.
1000 Kelvin has been quietly developing and testing its product, but it feels it has reached maturity to scale up. The company is now partnering with Autodesk to bring the AI-trained models into Autodesk Fusion and other designer-targeted software.
“We see Autodesk Fusion as the platform of innovators, engineers and designers. With the team of Autodesk, we integrated our AI models in our product to predict failures, automatically correct the design, and give it back to you. That new product from the partnership will democratize AM. With that, you can think of AM as a simple-to-use technology,” says Fergani.
More Ansys Coverage
More Autodesk Coverage
More Materialise Coverage
Subscribe to our FREE magazine,
FREE email newsletters or both!Latest News
About the Author

Kenneth Wong is Digital Engineering’s resident blogger and senior editor. Email him at [email protected] or share your thoughts on this article at digitaleng.news/facebook.
Follow DE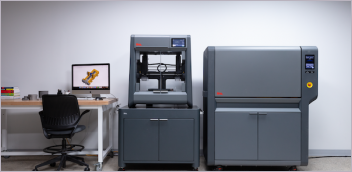
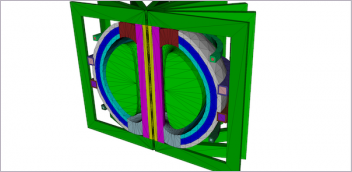
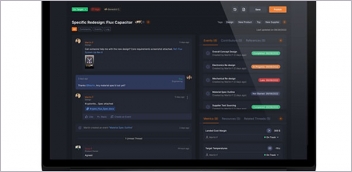
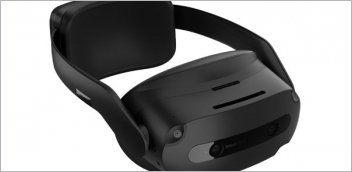